Where Are the Women in AI?
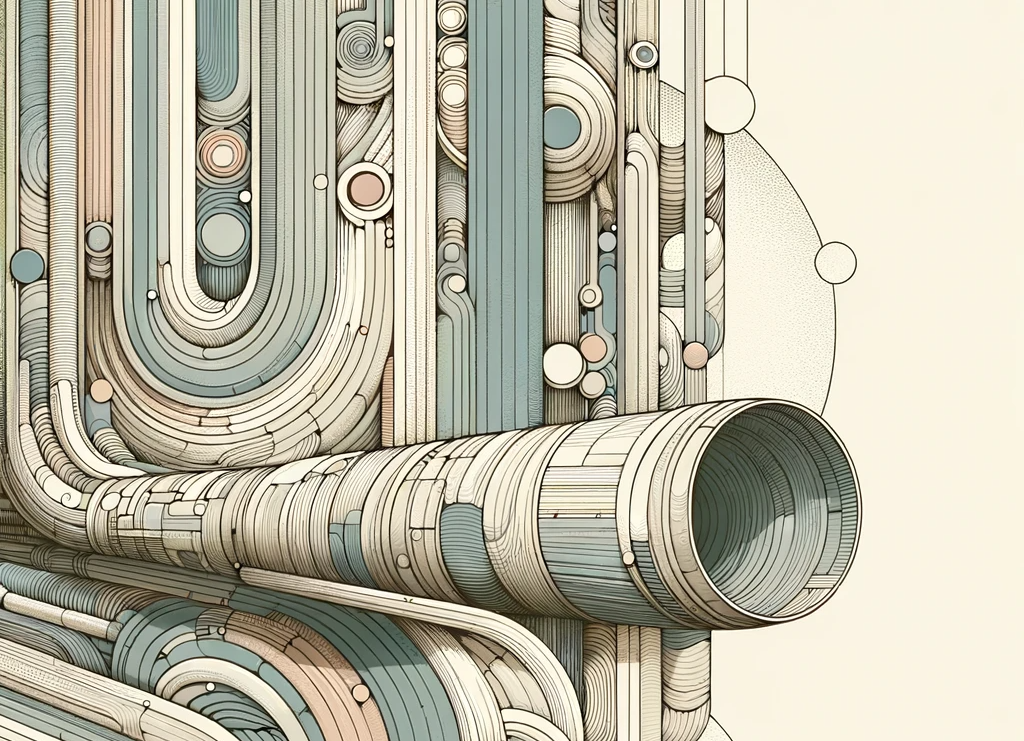
The drama at OpenAI has made it clear that we’re in the first season of a long-running reality show about the future of AI. If we’re signed up for episodes of mudslinging and backroom dealing, we may as well familiarize ourselves with the cast of characters.
Luckily, The New York Times recently published a list of the “who’s who” of AI futurists and their tech billionaire funders. Notably missing are nearly all of the prominent researchers in the field. Also notably missing are any women.
So the list is bad, but correcting it to include the real leaders in the field will do little to solve the diversity problem. Many people have called out the omission of Fei-Fei Li, a female pioneer in computer vision, but the other obvious omissions are, unfortunately, more men.
Where are all the women in AI?
They aren’t hiding, there just aren’t many of them. McKinsey found that women represent 27% of employees on “AI focused teams” and Deloitte estimated that women hold 26% of “data and AI positions.” These numbers seem high to me, but even if we’re being conservative, it’s clear the field is dominated by men.
A common defense of poor diversity numbers in the tech industry is to blame the pipeline: Our company would LOVE to hire a diverse workforce, but there are simply not enough underrepresented candidates who PASS THE BAR. As a hiring manager for software engineering roles, I’ve found that many strong candidates from non-traditional backgrounds are disqualified based on skills they could easily learn or skills that aren’t needed for the job. For all the talk of “hiring for the slope, not the y-intercept,” few engineering hiring managers put the mantra into practice.
That said, AI really does have a pipeline problem.
Something important to understand about AI: Nearly all of the practitioners have PhDs. Until recently, the fields that make up what we now call AI were small, insular academic communities, and the topics were rarely taught at an undergraduate level. People who “work in AI” are people who earned PhDs in those academic areas and are now professors at universities, researchers at industry labs or — increasingly — former academics who run AI startups. If you work in AI and have any level of seniority, you almost certainly went through the same narrow pipeline.
This is where I come into the story. I left the machine learning pipeline in the early 2010s. I don’t have any bombshell accusations, but I have some stories.
In my second year of grad school, I attended a workshop run by the Women in Machine Learning group. The workshop was held in a small room at the convention center that was hosting the field’s largest annual conference. Roughly 50 of us spent the day together presenting our work and by the time I settled in for the closing Q&A, I was feeling more confident about making it through the general conference. The senior women on the panel answered our questions for half an hour until one student asked for advice on how to become a successful professor like themselves. I can’t remember who answered, but she was direct. “If I had known what I know now, I would have never pursued this career,” she told us. “It’s a boys club out there, good luck.”
With that, the workshop ended and we walked into the convention hall filled with hundreds of research posters and thousands of men jockeying (sometimes physically!) to get facetime with the authors. The few friendly faces I knew quickly vanished and I was left to fend for myself.
That conference is called Neural Information Processing Systems and it was known by the acronym NIPS for thirty years. In 2018, several members of the community suggested the name be changed to something that didn’t sound like a female body part. A culture war ensued, fought on message boards and over Twitter. Many men declared that they never associated NIPS with nipples until the women suggested it. At some point Stephen Pinker weighed in with the unhelpful suggestion that “society should not endorse the idea that the concept of nipples is sexist.”
In October of that year, the NIPS board of trustees announced that they were not going to change the name. They had surveyed the community and the majority of members were happy with the status quo. Only 28% of the 2,270 male identifying survey participants and 44% of the 294 female identifying participants wanted the name change. The board did not comment on the even more shocking statistic that only 13% of their community identified as female.
There was backlash to the backlash and eventually the acronym was changed to NeurIPS. One artifact of the debate is an excellent paper titled, What’s in a name? The need to nip NIPS. I reread it recently and learned new details about the era. Apparently Elon Musk had made inappropriate jokes about the acronym on stage, some conference participants had worn lewd t-shirts, and someone had held a pre-conference event named TITS.
So there you have the pipeline problem in AI. And there’s data to back it up. According to the Stanford Artificial Intelligence Index, women represented only 18% of PhD graduates in machine learning when I was in graduate school. Ten years later, that number has only gone up to 21%.
I couldn't find a breakdown of AI PhD graduates by ethnicity, but here are the numbers across all CS PhD graduates. In 2021, only 4% of people graduating with PhDs in the field identified as Black and 5% identified as Hispanic. Environments that are not friendly to women tend to also be unfriendly to anyone from an underrepresented group.

Graduate students who don't like the culture of the field can stick around and try to change it, but they risk becoming jaded and, truthfully, very unhappy. The other option is to leave. I left and I have since found many interesting problems to work on outside of AI, but I know that there’s no way back into the club. There is one club, populated by one pipeline, and you have to stick it out to join.
0 Comments
Sign in or become a Machines on Paper member to join the conversation.
Just enter your email below to get a log in link.